Vol 20 (2021) Machine Intelligences
In Other Words:
Smart Compose and the Consequences of Writing in the Age of AI
Crystal Chokshi
If you use Gmail, like approximately 1.5 billion other people on the planet, you probably know what it means to press tab. ‘Tab’ is shorthand for interacting with Google’s new artificial intelligence (AI), which periodically offers predictions for your text; to tab is to accept them. Called Smart Compose, the AI’s objective is, ostensibly, quite simple: make quick predictions for the next word a user is likely to type.1
But Smart Compose’s function goes beyond making writing faster and easier, as its campaign promises. In this paper, I conceptualize the AI’s function in terms vastly different from the ones Google—and users such as those quoted below (Figure 1)—deploy to position it. Thinking through the AI with a data-colonialism frame as recently advanced by Nick Couldry and Ulises A. Mejias, I argue that Smart Compose is not only the helpful companion that the technology company purports it to be and that users seem to have embraced. It is also a means for Google freely to mine and monetize material that is more valuable to the company than anything else: words.
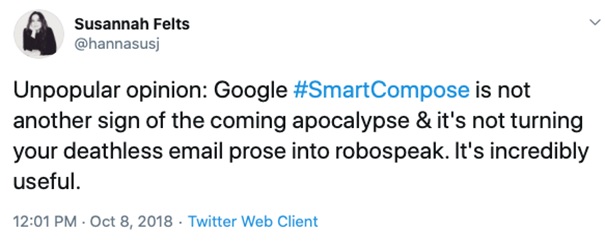
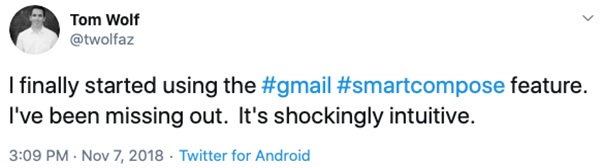
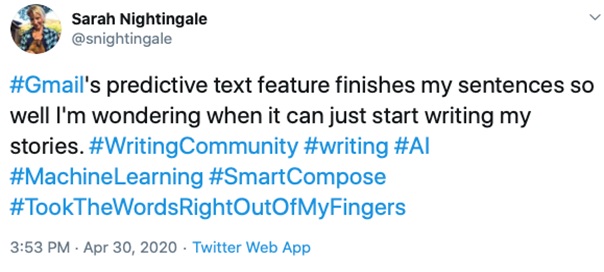
This argument will seem commonsensical to scholars familiar with debates surrounding Google and privacy. For instance, political economy scholars have pointed out Google’s exploitation of data entered into its various platforms (Fuchs 2011; Noble 2018; Mansell & Steinmueller 2020). And privacy scholars will be unsurprised that I position Smart Compose as another exploitative technology in Google’s repertoire. But the implications of my argument here go beyond privacy. Building on the work of linguistic capitalism scholars, who describe the new linguistic economy instantiated by Google, I argue that Smart Compose and technologies like it reconceptualize what it means to write, making the communicative function of writing secondary to its function as a commodifying act.
In this paper, I lean into debates and concepts surrounding media as extractive technology as a way into other debates altogether: the history, technologies, and consequences of writing. Combining scholarly work on the topics of critical media studies, critical algorithm studies, platform studies, and linguistic capitalism, I contextualize the current moment in this history, elaborating how platforms and AI shift ‘the semantic coordinates’ (Striphas 2015: 398) of what it means to write.
To trace this shift, I describe the conditions that enable it. I begin by introducing the concept of the calculating public, establishing it as an important precondition for data colonialism to function. I then discuss data colonialism in more detail, explaining how it is central to a critical understanding of Smart Compose and word-prediction technologies, more broadly. Finally, I conclude that applying a data-colonialism framework to Smart Compose illuminates the new consequences of writing in a platform society.
The production of a calculating public
In popular debates about word-prediction AI, one question tends to eclipse all of the others: what are its effects? A common response to predictive technologies is that their artificial intelligence will diminish our own. This sentiment is succinctly put by John Seabrook, writing about Smart Compose for The New York Times: ‘If I allowed [Google’s] algorithm to navigate to the end of my sentences how long would it be before the machine started thinking for me?’ (2019: para. 3). Seabrook epitomizes a techno-moral panic over human-machine entanglements, or what popular culture depicts as the slippery-slope of AI—not only might it, like the internet, make us stupid (Carr 2008), but it might also begin to think for us, perhaps even overtake us, launching us into the realm of the posthuman, where consciousness is merely a ‘minor side show’ (Hayles 1999: 3).
This kind of thinking, however, is reminiscent of debates about pernicious effects that have surrounded many once-contentious technologies, including the printing press, which was said to be especially dangerous to the health of female readers (Johns 1998); the carte de visite, suspected of degrading social respectability in Victorian social circles (Rudd 2017); the internet, charged with promoting promiscuity, anti-community, and hate (Krotoski 2014); and social media, accused of ‘mobiliz[ing] animosity toward common enemies and transform[ing] uneasy concern into full-blown panic’ (Walsh 2020: 6). While each of these individual ‘panics’ has served an important sociological function, communicating the values and ideologies of particular peoples at particular times, a critical-media approach to thinking through technology requires a different conceptual lens. As W.J.T. Mitchell once put it, ‘the “shock of the new” is as old as the hills, and needs to be kept in perspective’ (2005: 212-213).
Debating the effects of technologies as they are currently deployed has two consequences. First, it takes their design for granted. Second, and crucially, it forecloses discussion of a critical question posed by Siva Vaidyanathan, a scholar whose eyes are also trained on Google: ‘What are the cultural and economic assumptions that influence the ways a technology works in the world?’ (2011: 16). Perhaps the most crucial assumption built into the Smart Compose campaign is this one: writing is a waste of time. Gmail’s blog post introducing the AI in May 2018 emphasizes the importance of staying connected to friends and family but laments the time required to doing so: ‘Email makes it easy to share information with just about anyone—friends, colleagues and family—but drafting a message can take some time’ (Lambert 2018: para. 1). Thus, says Gmail product manager Paul Lambert, ‘Today, we’re announcing Smart Compose, a new feature powered by artificial intelligence, to help you draft emails from scratch, faster’ (2018: para. 1). Several months later, this key message would be reiterated by senior product manager Tom Holman who, on Gmail’s fifteenth birthday, pointed out that in its maturity Gmail was now ‘more assistive’ because of ‘Smart Compose, an AI-powered feature that helps you write emails quicker’ (2019: para. 6). Elsewhere, Google’s messaging is less subtle, deploying language that labels email ‘a real pain’ (Corrado 2015: para. 2), ‘time-consuming’ (Kannan et. al. 2016: 1), and ‘repetitive idiomatic writing’ (Chen et al. 2019: 2). While this messaging does not call into question the necessity of writing email, taking for granted email’s function as connection-builder and connection-keeper amongst distant family and friends, it does suggest that the time required to do so is not time well-spent. The problem, Google employees write, stems from email’s consisting of repetitive actions (Chen 2019; Lambert, 2018), which the AI is designed to eliminate.
By describing writing as a set of patterns and repetitions, Google positions it as a task that can easily and naturally be outsourced to AI. In doing so, the company invokes the discourse of self-optimization. Individuals and societies who value self-optimization are relentlessly in pursuit of the most efficient ways to accomplish tasks in order to be productive (Bloom 2018). Productivity, in turn, is often assessed quantitatively (Stepanchuk 2017). Thus, the discourse of self-optimization lays the groundwork for datafication, which ‘refers to the ability of networked platforms to render into data many aspects of the world that have never been quantified before’ (van Dijck et al. 2018: 33). Both discourses—self-optimization and datafication —are particularly visible in a Tweet posted by Gmail in October 2018 announcing that Smart Compose was ‘sav[ing] people from typing over 1B[illion] characters each week—that’s enough to fill the pages of 1,000 copies of Lord of the Rings’ (Holman 2019: para. 6). Several months later, this number would be updated, noting that Smart Compose was realizing users a ‘savings’ of two billion characters a week (Pichai 2018). These statistics are inventions constrained by what computers are most capable of: counting. They are numbers that merely pose as relevant metrics. Nonetheless, they dangle in front of users the promise of betterment. Not only do they present the potential savings for users in numbers (i.e. billions) that connote the epitome of achievement (consider our culture’s reverence for billionaires), but they also imagine the literal weight and volume of two billion characters saved: 2,000 copies of Tolkien’s Lord of the Rings. According to the logic of this post, not adopting Smart Compose would be a blatant misplacement of time and energy, and misplacing time and energy violates the construction of the optimized, datafied self.
These particular acts—positing an activity as necessary but inefficient, invoking the discourses of self-optimization and datafication, and, finally, diverting users’ attention to statistics and measures that previously did not exist—constitute chief characteristics of what I call the production of a calculating public. I borrow the bulk of this phrase from Tarleton Gillespie. He calls the ‘production of a calculated public’ those processes and practices that ‘invok[e] and clai[m] to know a public with which we are invited to feel an affinity’ (2014: 188). For example, Gillespie argues that when, after completing my Amazon purchase, I see a message that reads, ‘Users like you also bought…’, I am invited to think of a virtual community of which I am a part. In some ways, Smart Compose is illustrative of Gillespie’s concept. Claiming to have memorized ‘only common phrases used by multiple users around the world’ (Wu, 2018: para. 12), the AI invites users to think of themselves as a global community woven together by virtue of a shared vocabulary. But in modulating Gillespie’s language, I also modulate the concept. While a calculating public may be invited to think of shared interests or networks, its focus is sharply oriented to self-improvement and self-optimization.
The production of a calculating public not only involves diverting user attention to quantifications where they previously did not exist. It also involves diverting this attention to quantifications that may not even necessarily be relevant. In Gillespie’s example surrounding Amazon recommendations, ‘the population on which [Amazon] bases these recommendations is not transparent, and is certainly not coterminous with its entire customer base’ (2014: 188). Similarly, Smart Compose’s quantifications have value only in the context of a vague public. Its predictions have been trained on an unspecified corpus produced by an unspecified population. While billions of words collected over the course of one year have tuned Smart Compose’s sensibilities (Seabrook 2019), we do not know exactly which words—or whose—were collectable, categorized, or excluded. Who, then, are among the users seeing the two-billion-character savings?
To further challenge the relevance and significance of this metric, I offer up some simple math: two billion characters saved weekly by 1.5 billion users is a savings of less than two characters per user each week. To be clear, two characters is the equivalent of ‘Lo’, nowhere near close to the full title of Lord of the Rings, never mind 2,000 copies of the text. In terms of time, eliminating the need to type two characters translates into a savings of roughly 200 milliseconds (Wu 2018). This is not enough even to read Tolkien’s title. Of course, my math rests on several assumptions, including Smart Compose’s rate of adoption (the feature can be shut off), but the math is the minutiae. What these numbers underscore is the precarity of Smart Compose’s benefit statements, and they emphasize that the production of a calculating public means asking users to buy into quantifications whose value is questionable to the very users it claims to support.
Data colonialism
If there is uncertainty around the benefits that Smart Compose provides, it is only compounded by the AI’s origin story. In the research paper that accompanied Smart Compose’s launch, the engineering team described Smart Compose’s purpose in the following way:
E-mail continues to be a [sic] ubiquitous and growing form of communication all over the world, with an estimated 3.8 billion users sending 281 billion e-mails daily. Improving the user experience by simplifying the writing process is a top priority of major e-mail service providers like Gmail… In this paper, we introduce Smart Compose, a system for providing real-time, interactive suggestions to help users compose messages quickly and with confidence in Gmail. Smart Compose helps by cutting back on repetitive idiomatic writing via providing immediate context-dependent suggestions… Smart Compose assists with composing new messages from scratch and provides much richer and more diverse suggestions along the way, making e-mail writing a more delightful experience (Chen et al. 2019: 1-2).
(Chen et al. 2019: 1-2)
There is an unresolved tension between the first sentence and the last. The authors open with a statement about the expansive market in which Gmail, one of Google’s major lines of business, resides. However, the arc of market opportunity is not traced; instead, they suggest that the AI’s objective is merely to support users—to inspire more confidence and to create more delightful experiences. This seems remarkably quaint for a company that ‘instrumentalizes all users and all of their data for creating profit’ (Fuchs 2011: 36). Similar to how ‘Mantras like [Facebook’s] “making the world more open and connected”… conceal actual contributions to public value’ (van Dijck et al 2018: 149), my contention is that Gmail’s purported desire to be in service of users seems to mask something else. This ‘something else’ is the true value—and intended use—of users’ words.
The key to understanding what is concealed, and how the concealment occurs, is a framework called data colonialism. Articulated by Nick Couldry and Ulises A. Mejias, data colonialism is ‘a new form of colonialism, normalizing the exploitation of human beings through data, just as historic colonialism appropriated territory and resources and ruled subjects for profit’ (2019: 336). It is predicated on the existence of what Couldry and Mejias call ‘data relations’, a ‘new type of human relations which enable the extraction of data for commodification’ (2019: 337). In other words, because social life is now carried out in so many ways in digital spaces, visible to surveillant apparatuses, human life itself becomes an open resource, ripe for extraction (Couldry 2020).
The notion of data relations is an idea that builds on a body of work that views media’s extractive dimension as critical to understanding media itself. In the late 1970s, Dallas W. Smythe conceptualized the ‘audience commodity’ (1977: 1) as ‘the most central feature of the communications system’ (Dolber 2016: 749). In doing so, Smythe turned on its head the notion of what media is all about—not content, he proposed, but rather the production of demand for consumer goods. The terms ‘prosumer’ and ‘produser’, introduced respectively by Alvin Toffler (1980) and Axel Bruns (2008), further built on the idea that media, for providing a service, demands something in return: while using or consuming media, users also produce activity, data, and, therefore, value for media companies. Marxist critic Christian Fuchs, in turn, has relied on this triad of concepts to theorize (and criticize) Google. The crux of Fuchs’ indictment is ‘not the technologies provided by Google, but the capitalist relations of production, in which these technologies are organized. The problem is that Google for providing its services necessarily has to exploit users and to engage in the surveillance and commodification of user-oriented data’ (2011: 46). What Fuchs describes is what Joseph Turow and Nick Couldry call ‘media as data extraction’ (2018), or what Jathan Sadowski calls the ‘data imperative’, which ‘demands the extraction of all data, from all sources, by any means possible’ (2019: 2). These activities are carried out against, and depend on, a backdrop of surveillance capitalism wherein citizens are subject to the constant monitoring and tracking of their behaviors in order for companies ‘to produce new markets of behavioral prediction and modification’ (Zuboff, 2015: 75).
Couldry and Mejias note that data colonialism rests on several systematic assumptions. Not least of all, personal data ‘must first be treated as a natural resource, a resource that is just there’ (Couldry & Mejias 2019: 339). Just as importantly:
Jason Moore (2015) argues that capitalism historically depended on the availability of cheap nature: natural resources that are abundant, easy to appropriate from their rightful owners, and whose depletion is seen as unproblematic… So too with what we now call ‘personal data’.
(Couldry & Mejias 2019: 339)
In other words, the ‘just there-ness’ of personal data is a deliberate positioning consistent with the positioning that has facilitated, for example, the forestry, mining, and fishing industries. And it is also, more troublingly, consistent with the positioning that gave rise—and continues to give rise—to systems of slavery, imperial domination, and myriad forms of discrimination and oppression. Not just data but human life itself has been positioned as cheap nature, subject to exploitation and forcible removal.
These colonial histories and logics are at play in designs of the digital future that technologies such as Smart Compose play out. Smart Compose’s marketing materials take for granted the ‘just there-ness’ of Gmail users’ personal data: their words. Neither blog posts nor Tweets meditate on the ethics of recording and machine-reading the personal correspondence of an untold number of Gmail’s 1.5 billion users for the purpose of developing its own intellectual property (IP). Nor do they seek the user’s input or consent. Rather, the messaging Google promotes is more like this:
I get a lot of email, and I often peek at it on the go with my phone. But replying to email on mobile is a real pain, even for short replies. What if there were a system that could automatically determine if an email was answerable with a short reply, and compose a few suitable responses that I could edit or send with just a tap? Some months ago, Bálint Miklós from the Gmail team asked me if such a thing might be possible. I said it sounded too much like passing the Turing Test to get our hopes up… but having collaborated before on machine learning improvements to spam detection and email categorization, we thought we’d give it a try.
(Corrado 2015: para. 2-3)
Blog posts such as Google senior research scientist Greg Corrado’s (which here describes Smart Compose’s predecessor, Smart Reply) use first-person pronouns, anecdotes, and the identification of a common or universal foe (time, in this case) in the company’s effort to be relatable. Although no doubt vetted, if not written, by senior marketing and communications staff, posts are labelled with the names of mid-level employees, such as ‘product manager’ or ‘principal engineer’, adding an extra dimension to relatability. Similar to ‘Stars are just like us!’ pages in People or Us Magazine, these posts show Google employees in everyday life, reporting on everyday challenges and experimenting their way through them. That blog posts are sometimes framed by what-if questions prompted by candid water-cooler conversation lends even more credence to the narrative of challenge, experimentation, and overcoming. What this framing does better than anything is sidestep questions around data’s rightful ownership.
It also downplays the value of users’ words. Corrado speaks of writing in terms of its being ‘a real pain’—routine, repetitive, and low-reward. Not only does this positioning lay the groundwork, as aforementioned, for invoking the discourses of self-optimization and datafication, but it also performs the work of transforming billions of Gmail users’ acts of writing into cheap nature. If users are to believe the campaign surrounding Smart Compose, writing is an activity bound up with both waste and excess: words on which users should not waste time and composition practices that are extraneous to communicative tasks at hand. Running invisibly in the background, watching and recording what users type, Smart Compose becomes a benevolent presence, making predictions for words users wish they were not writing in the first place thus, eliminating yet another mundane facet of modern life.
Colonizing words
There is a paradox, however, at the heart of the Smart Compose campaign. It is located in the very statistic that Google uses to allege Smart Compose’s benefits (Figure 2). In order to assert that Smart Compose saves users from typing more than two billion characters each week, Google must know which predictions are deployed. That is, in order to track what has not been typed, Google must be tracking what is. This means that while Smart Compose’s aim is to divest users of words, it also collects them, and it is heavily invested in information about them. The paradox, then, is this: while Google’s marketing derides writing as valueless, words, when it comes to Smart Compose, are invaluable.
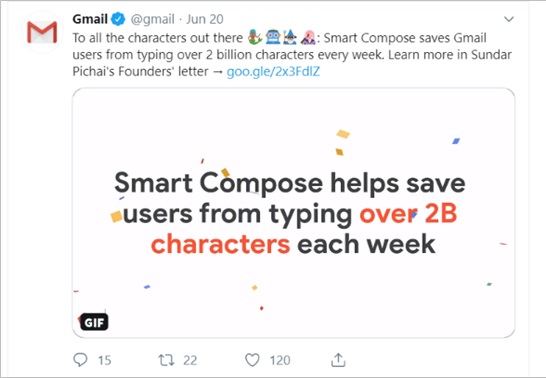
Words, indeed, are incredibly valuable assets across Google’s platforms. They are directly linked to dollars and cents. This is because of Google Ads (formerly Google AdWords), the extant platform through which Google commoditizes search (Bruno 2002; Kaplan 2014; Noble 2018; Thornton 2018). For example, let us say that I have a Calgary-based window-washing company, and I want my website to appear in search results for ‘window washing Calgary’. To do this, I would use keywords—windows, washing, cleaning, Calgary—as much as possible within the website so that it stands a higher chance of appearing on the first page of search results. This is what industry calls ‘search engine optimization’. I would also bid on keywords—for instance, ‘window’, ‘washing’, and ‘Calgary’—through the Google Ads platform. ‘When a Google user searches the Web using one or more of those keywords, the ad appears on the [search engine results page] in a sidebar. The advertiser pays Google every time a user clicks on the ad’ (Strickland & Donovan 2019: para. 3). In 2019, Google Ads generated the majority of Google’s $162 billion revenue (Rosenberg 2020).
The idea of words as potentially profitable data has been explored under various names by various scholars since the 1970s (Thornton 2019). Frédéric Kaplan, however, is the first to have coined the term ‘linguistic capitalism’ (Thornton 2018), which describes Google Ads’ relationship to words. By having a large user base ‘enter[ing] ever-larger numbers of search queries’ (Kaplan 2014: 58), ‘Google has extended capitalism to language, transforming linguistic capital into money’ (59). The result, writes Kaplan, is that ‘words and expressions… become commodities’ (59). Consequently, this commoditization engenders:
the shrinking of the creative vocabulary of digital language in favor of the most popular keywords… [and] the rehashing of existing content, rather than anything new, and although it could be said that SEO is in itself a creative industry, unless it is also economically lucrative, there is little value to Google in original, or creative language.
(Thornton 2018: 423)
Most importantly, Pip Thornton observes that uncommon words have little capital in Google’s system. It is the most commonly-used and -sought words that Google wants to see circulate, as they stand to earn Google the most money.
That Google makes money based on the circulation of commonly-used words is a fact that inflects the function of autocomplete and autocorrect. While autocomplete and autocorrect appear to be about being intuitive and useful, just like Gmail’s brand promise, they serve another purpose altogether: ‘When Google’s autocompletion service transforms on the fly a misspelled word, it does more than offer a service. It transforms linguistic material without value (not much bidding on misspelled words) into a potentially profitable economic resource’ (Kaplan, 2014: 59). For instance, if I mistype ‘free local activities’ as ‘fere local activities’, and Google offers an autocorrect that transforms my search into a more common phrase, Google stands to earn much more (Figure 3).
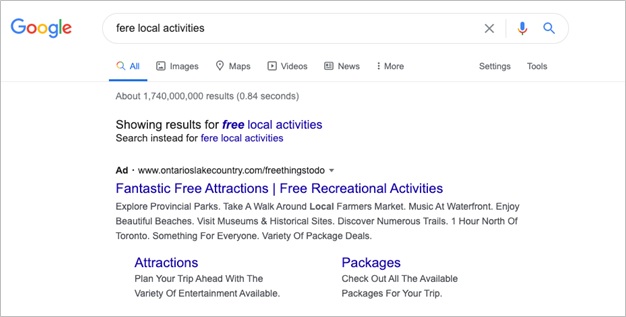
By extending the work of linguistic capitalism scholars, I trouble Smart Compose’s alleged desire merely ‘to help users compose messages quickly and with confidence in Gmail’ and to ‘mak[e] e-mail writing a more delightful experience’ (Chen et al. 2019: 1-2). If autocorrect and autocomplete transform natural language into economically-exploitable language, then so, too, does Smart Compose. In fact, the prediction of ‘regular’ expression is Smart Compose’s explicit aim, with engineers stating that ‘only common phrases used by multiple users are memorized by our model’ (Wu 2018: para. 12). Simply put, Smart Compose predicts common phrases, and common phrases, as Bruno (2002), Kaplan (2014), and Thornton (2018; 2019) have noted, are lucrative.
Common phrases stand to be lucrative in Gmail because Gmail, like Google’s other platforms, is supported by advertising revenue. Gmail Ads function similarly to Google Ads: advertisers can bid on keywords, targeting ads to users who employ them. Given that Google has data surrounding which of Smart Compose’s predictions are deployed—a fact, as aforementioned, suggested by Smart Compose’s publicized metrics—the company has the information it needs to better forecast and grow advertising revenue: it knows which of the predictions in its model are the most common and, thus, the keywords for which it can charge more. In other words, Smart Compose creates a dataset that Google can provide to prospective advertisers.2
While Google reported in 2017 that it was discontinuing its reading of individual email messages for the purpose of serving up personalized ads (Greene 2017), this does not mean Google has discontinued scanning or discontinued personalized advertising altogether. As one writer has pointed out:
Does [the discontinuation of email-scanning] mean that Google will stop looking at your email? Not exactly. The company has also long been scanning Gmail accounts for other reasons, and in fact increased product personalization based on the emails you get over the years. The Google app on your phone, for example, knows when your next flight is leaving, and whether or not it has been delayed, based on emails you get from airlines and travel booking sites. Similarly, Google Calendar has begun to automatically add restaurant reservations and similar events to your schedule based on the emails you are getting. Google also has for some time automatically scanned emails for links to potentially fraudulent sites, as well as to filter out spam.
(Roettgers 2017: paras. 6-7)
Gmail also explicitly states that it scans email for the purpose of fine-tuning Smart Compose’s predictions. On the occasion of Gmail’s fifteenth birthday, Google senior product manager Tom Holman wrote that:
Smart Compose is also getting, well, smarter. It will personalize suggestions for you, so if you prefer saying “Ahoy,” or “Ello, mate” in your greetings, Smart Compose will suggest just that. It can also suggest a subject line based on the email you’ve written.
(2019: para. 7)
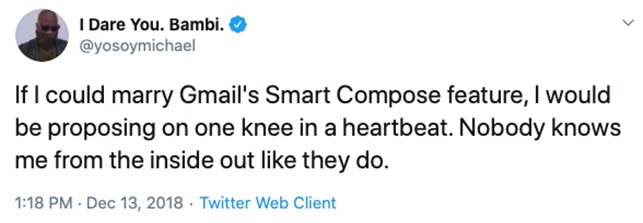
What Holman reveals is that Smart Compose is constantly machine-reading users’ email in order to update its prediction model. This, of course, is how machine-learning functions: a model requires feedback in order to become ‘smarter’. Because Holman does not discuss any action that a user must take in order to transmit feedback, we must assume that Gmail data—i.e. words—are always being communicated to the Smart Compose database. While Google might not be scanning individual email, then, for the purpose of personalizing ads in the way it used to, it is nonetheless scanning email for the purpose of better ‘knowing’ its users (and users are feeling known—see Figure 4). This information, even if anonymized, remains valuable to prospective advertisers, who, in spite of Google’s updated advertising model, bid on keywords for their Gmail Ads campaign in the effort to target relevant audiences (Google Ads Help 2020a; Google Ads Help 2020b; Google Ads Help 2020c; Gmail Help 2020). In other words, while Gmail’s scanning, advertising, and personalization practices may have shifted, they have most certainly not been abandoned.
Re: Writing
In this paper, I have offered a conceptualization of Smart Compose that runs counter to mainstream narratives about it. Stressing the tension between what users imagine word-prediction AI achieves and what it actually carries out, I have argued that Smart Compose is not merely a means to engineer a more ‘delightful’ email experience. Building on the work of linguistic capitalism scholars, I demonstrate how it supports the forecasting and growth of advertising revenue for the media behemoth that is Google. Smart Compose does this by playing a role similar to the one scholars have discussed in relation to autocorrect and autocomplete: these technologies funnel linguistic expression—words and search terms—into language that has economic value to Google and its customers. In this way, the AI is best understood as an extractive technology. While marketing campaigns claim that the technology exists simply to help users write emails ‘better’ and ‘faster’, Smart Compose monitors and commodifies users’ data in order to produce demand for consumer goods.
Vital to this model’s operation is the assumption that acts of writing are repetitive, time-consuming, and inefficient. Positioned this way by marketing campaigns, words appear to have little value. Thus, Gmail users are persuaded to become a calculating public: a community who readily accepts datafication, quantification and opportunities for self- optimization. But through the framework of data colonialism, the trade-off implicit in this stance emerges. Through this lens, words are revealed to be the very thing that Google must downplay in order to uphold its market share. Words are simultaneously ‘cheap nature’ and invaluable resources. They are things that Google urges users not to invest in while at the same time they are what Google, across its platforms, is invested in more heavily than anything else. The sum total is yet another paradox in the platform ecosystem, which is already rife with them (Mansell 2012; van Dijck et al. 2018).
But in addition to instantiating another paradox in the platform ecosystem, Smart Compose carries another major implication: it enacts a shift in what it means to write, if writing is understood performatively. A performative perspective holds that ‘what something is has to be understood in terms of what it does’ (Drucker 2013: para. 4). What Smart Compose does is put commodification at the heart of writing itself. When users write with Smart Compose, we are not engaged only in the process of transmitting a message from A to B. We are simultaneously deploying data from, and generating data for, the Smart Compose database. Because this database, as I have argued, might ultimately serve Google’s bottom line, we are also, if only unwittingly, contributing to it. In this paradigm, writing does things beyond communication: it becomes a vehicle for data capture, data generation, and wealth accumulation for a massive technology company.
In the age of platforms and AI, then, the semantic coordinates of what it means ‘to write’ are shifting. ‘To write’ with Smart Compose is to deploy words that have taken on the shape and scale of big data. It is to consent to having these acts of deployment become, in turn, more data, as Smart Compose’s predictive model is continuously updated by the billions of deployment choices made by its billion-plus users. And it is ultimately to participate in the ‘economic game’ whose goal is ‘to develop intimate and sustainable linguistic relationships with the largest possible number of users in order to model linguistic change accurately and mediate linguistic expression systematically’ (Kaplan 2014: 60). Because this intimate linguistic relationship occurs without user consent, without discussion around the ethics of recording and machine-reading the personal correspondence of an untold number of Gmail’s 1.5 billion users for the purpose of developing its own IP, and without acknowledging the commercial purposes that Smart Compose might ultimately serve, to write with Smart Compose is not only to feel known and loved, as many users have reported, by AI. It is also—as so many lovers, at one time or another, find themselves—to be duped.
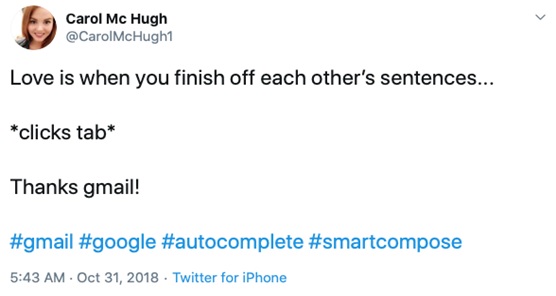
Notes
1. Smart Compose also appears in Google Docs (for users with enterprise accounts), but I deal here exclusively with Gmail, as Google has discussed and marketed the AI chiefly in relation to Gmail.
2. Admittedly, this discussion is not entirely free of speculation. Zachary C. Lipton and Jacob Steinhardt (2019) observe that a problematic trend in machine-learning scholarship is the insufficient differentiation between speculative and explanatory discussion. Speculative discussion involves “exploration predicated on intuitions that have yet to coalesce into crisp formal representations” (p. 46). To be clear, my discussion here falls somewhat in this camp, as I am triangulating based on information published by various Google sources, and I am extrapolating the potentialities of Smart Compose based on the political-economic analyses carried out by other critical scholars.
Acknowledgements
This research is supported by an Izaak Walton Killam Memorial Scholarship and a Social Sciences and Humanities Research Council Doctoral Fellowship, for which I am grateful. I also extend sincere thanks to Dr. Mél Hogan, Dr. Annie Rudd, Tessa Brown, Emma Kilburn-Smith, Andrew Kacey Thomas, and the anonymous reviewers whose thoughtful feedback made this article something so much better than I could have achieved on my own.
References
Bloom, P. (2018) “Radical Optimization: On ‘Desperately Seeking Self-Improvement’”, Los Angeles Review of Books (March 14).
Bruno, C. (2002) “The Google AdWords Happening”, Iterature. http://www.iterature.com/adwords/.
Bruns, A. (2008) Blogs, Wikipedia, Second Life, and Beyond: From Production to Produsage. New York: Peter Lang.
Carr, N. (2008) “Is Google Making Us Stupid?”, The Atlantic (June/July 2008).
Chen, M. et al. (2019) “Gmail Smart Compose: Real-Time Assisted Writing”. KDD ’19: Proceedings of the 25th ACM SIGKDD International Conference on Knowledge Discovery & Data Mining. (July): 2287–2295.
Corrado, G. (2015) “Computer, Respond to This Email”, Google AI Blog (November 3): https://ai.googleblog.com/2015/11/computer-respond-to-this-email.html.
Couldry, N. (2020) “Episode 3: Data Colonialism w/Professor Nick Couldry”, Soundcloud. https://soundcloud.com/in_beta/episode-3-data-colonialism.
Couldry, N. & Mejias, U. (2019) “Data Colonialism: Rethinking Big Data’s Relation to the Contemporary Subject”, Television & New Media 20. No. 4: 336-349.
Dolber, B. (2016) “Blindspots and Blurred Lines: Dallas Smythe, the Audience Commodity, and the Transformation of Labor in the Digital Age”, Sociology Compass 10. No. 9: 747-755.
Drucker, J. (2013) “Performative Materiality and Theoretical Approaches to Interface’, Digital Humanities Quarterly 7. No. 1.
Fuchs, C. (2011) “A Contribution to the Critique of the Political Economy of Google”, Fast Capitalism 8. No. 1: 31-50.
Gillespie, T. (2014) “The Relevance of Algorithms”, in Media Technologies: Essays on Communication, Materiality, and Society, (eds.) T. Gillespie et al. Cambridge: MIT Press.
Gmail Help. (2020) “How Gmail Ads Work”, Google. https://support.google.com/mail/answer/6603?hl=en.
Google Ads Help. (2020a) “Choose Keywords for Display Network Campaigns”, Google. https://support.google.com/google-ads/answer/2453986.
Google Ads Help. (2020b) “Optimize Display Network Ads And Campaigns”, Google. https://support.google.com/google-ads/answer/2549129?hl=en.
Google Ads Help. (2020c) “Show Your Gmail Ads to the Right People”, Google. https://support.google.com/google-ads/answer/7299965?hl=en.
Greene, D. (2017) “As G Suite Gains Traction in the Enterprise, G Suite’s Gmail and Consumer Gmail To More Closely Align”, The Keyword (June 23): https://blog.google/products/gmail/g-suite-gains-traction-in-the-enterprise-g-suites-gmail-and-consumer-gmail-to-more-closely-align/.
Hayles, N. K. (1999) How We Became Posthuman: Virtual Bodies in Cybernetics, Literature, and Informatics. Chicago: University of Chicago Press.
Holman, T. (2019) “Hitting Send on the Next 15 Years of Gmail”, The Keyword (April 1): https://www.blog.google/products/gmail/hitting-send-on-the-next-15-years-of-gmail/.
Johns, A. (1998) The Nature of the Book: Print and Knowledge in the Making. Chicago and London, UK: University of Chicago Press.
Kannan. A. et al. (2016) “Smart Reply: Automated Response Suggestion for Email”, KDD ’16: Proceedings of the 22nd ACM SIGKDD International Conference on Knowledge Discovery and Data Mining. (August): 955-964.
Kaplan, F. (2014) “Linguistic Capitalism and Algorithmic Mediation”, Representations 127. No. 1: 57-63.
Krotoski, A. (2014) “Inventing the Internet: Scapegoat, Sin Eater, and Trickster”, in Society and the Internet, (eds.) M. Graham & W. Dutton. Oxford: Oxford University Press.
Lambert, P. (2018) “SUBJECT: Write Emails Faster with Smart Compose in Gmail”, The Keyword (May 18): https://www.blog.google/products/gmail/subject-write-emails-faster-smart-compose-gmail/.
Lipton, Z. & Steinhardt, J. (2019) “Research for Practice: Troubling Trends in Machine-learning Scholarship”, Communications of the ACM 62. No. 6: 45-53.
Mansell, R. & Steinmueller, W. (2020) Advanced Introduction to Platform Economics. Cheltenham, UK and Northampton, MA: Edward Elgar Publishing.
Mitchell, W. (2005) What Do Pictures Want? Chicago: University of Chicago Press.
Noble, S. (2018) Algorithms of Oppression: How Search Engines Reinforce Racism. New York: NYU Press.
Pichai, S. (2018) “2018 Founders’ Letter”, Alphabet Investor Relations. https://abc.xyz/investor/founders-letters/2018/.
Roettgers, J. (2017) “Google Will Keep Reading Your Emails, Just Not for Ads”, Variety (June 23): https://variety.com/2017/digital/news/google-gmail-ads-emails-1202477321/.
Rosenberg, E. (2020) “How Google Makes Money”, Investopedia. https://www.investopedia.com/articles/investing/020515/business-google.asp.
Rudd, A. (2017) “Victorians Living in Public: Cartes de Visite as 19th-Century Social Media”, Photography and Culture 9. No. 3: 195-217.
Sadowski, J. (2019) “When Data is Capital: Datafication, Accumulation, and Extraction”, Big Data & Society. (January): https://doi.org/10.1177/2053951718820549.
Seabrook, J. (2019) “The Next Word: Where Will Predictive Text Take Us?”, The New Yorker (October 14).
Smythe, D. (1977) “Communications: Blindspot of Western Marxism”, Canadian Journal of Political and Social Theory 1. No. 3: 1-27.
Stepanchuk, Y. (2017) “Quantified Construction of Self: Numbers, Narratives and the Modern Individual”, IMS2017: Proceedings of the International Conference IMS-2017. (June): 28-36.
Strickland, J. & Donovan, J. (2019) “How Google Works”, How Stuff Works. https://computer.howstuffworks.com/internet/basics/google.htm.
Striphas, T. (2015) “Algorithmic Culture”, European Journal of Cultural Studies 18. No. 4-5: 395-412.
Thornton, P. (2019). “Language in the Age of Algorithmic Reproduction: A Critique of Linguistic Capitalism” (unpublished doctoral dissertation). Royal Holloway: University of London.
Thornton, P. (2018) “A Critique of Linguistic Capitalism: Provocation/Intervention”, GeoHumanities 4. No. 2: 417-437.
Toffler, A. (1980) The Third Wave. New York: William Morrow.
Turow, J. & Couldry, N. (2018) “Media as Data Extraction: Towards a New Map of a Transformed Communications Field”, Journal of Communication 68. No. 2: 415-423.
Vaidhyanathan, S. (2011) The Googlization of Everything (And Why We Should Worry). Berkeley and Los Angeles: University of California Press.
Van Dijck, J. et al. (2018) The Platform Society: Public Values in a Connective World. Oxford: Oxford University Press.
Walsh, J.P. (2020) “Social Media and Moral Panics: Assessing the Effects of Technological Change on Societal Reaction”, International Journal of Cultural Studies 23. No. 6: 840-859.
Wu, Y. (2018) “Smart Compose: Using Neural Networks to Help Write Emails”, Google AI Blog (May 16): https://ai.googleblog.com/2018/05/smart-compose-using-neural-networks-to.html.
Zuboff, S. (2015) “Big Other: Surveillance Capitalism and the Prospects of an Information Civilization”, Journal of Information Technology 30. No. 1: 75-89.